10-2024 |
New! Math-Vision is accepted in NeurIPS 2024 Datasets and Benchmarks Track! |
07-2024 |
New! We release SAM 2, a unified model for real-time, promptable video object segmentation. |
06-2024 |
We release Math-Vision, a benchmark for evaluating the mathematical reasoning abilities of LMMs. |
09-2023 |
JourneyDB is accepted in NeurIPS 2023 Datasets and Benchmarks Track! |
07-2023 |
We release JourneyDB, a large-scale benchmark for multimodal generative image understanding. |
05-2023 |
Starting my internship as a research scientist Intern at Meta AI (FAIR). |
09-2022 |
Our paper ST-Adapter on efficient image-to-video transfer learning is accepted to NeurIPS 2022. |
| | SAM 2: Segment Anything in Images and Videos Nikhila Ravi, Valentin Gabeur, Yuan-Ting Hu, Ronghang Hu, Chaitanya Ryali, Tengyu Ma, Haitham Khedr, Roman Rädle, Chloe Rolland, Laura Gustafson, Eric Mintun, Junting Pan, Kalyan Vasudev Alwala, Nicolas Carion,Chao-Yuan Wu Ross Girshick, Piotr Dollár, Christoph Feichtenhofer Arxiv, 2024 [paper] [website] [demo] [code] We present Segment Anything Model 2 (SAM 2 ), a foundation model towards solving promptable visual segmentation in images and videos. |
| ---------------------------------------------------------------------------------------------------------------------------------------------------------------------------------------------------------------------------------------------------------------------------------------------------------------------------------------------------------------------------------------------------------------------------------------------------------------------------------------------------------------------------------------------------------------------------------------------------------------------------------------------------------------------------------------------------------------------------------------------------------------------------------------------------------------------------------------------------------------- |
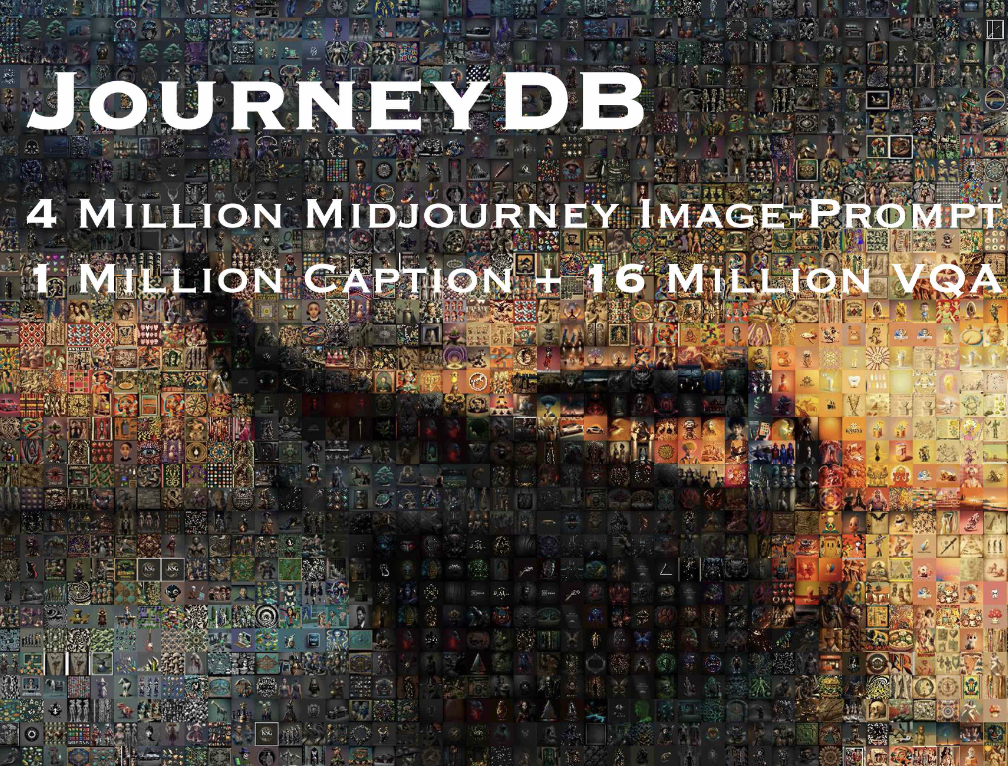 |
JourneyDB: A Benchmark for Generative Image Understanding Junting Pan*, Keqiang Sun*, Yunying Ge, Hao Li, Haodong Duan, Xiaoshi Wu, Renrui Zhang, Aojun Zhou, Zipeng Qin, Yi Wang, Jifeng Dai, Liming Wang, Yu Qiao, Hongsheng Li NeurIPS DB, 2023 [paper] [website] JourneyDB is a large-scale generated image understanding dataset that contains 4,4M high-resolution generated images, annotated with corresponding text prompt, image caption, and visual question answering. |
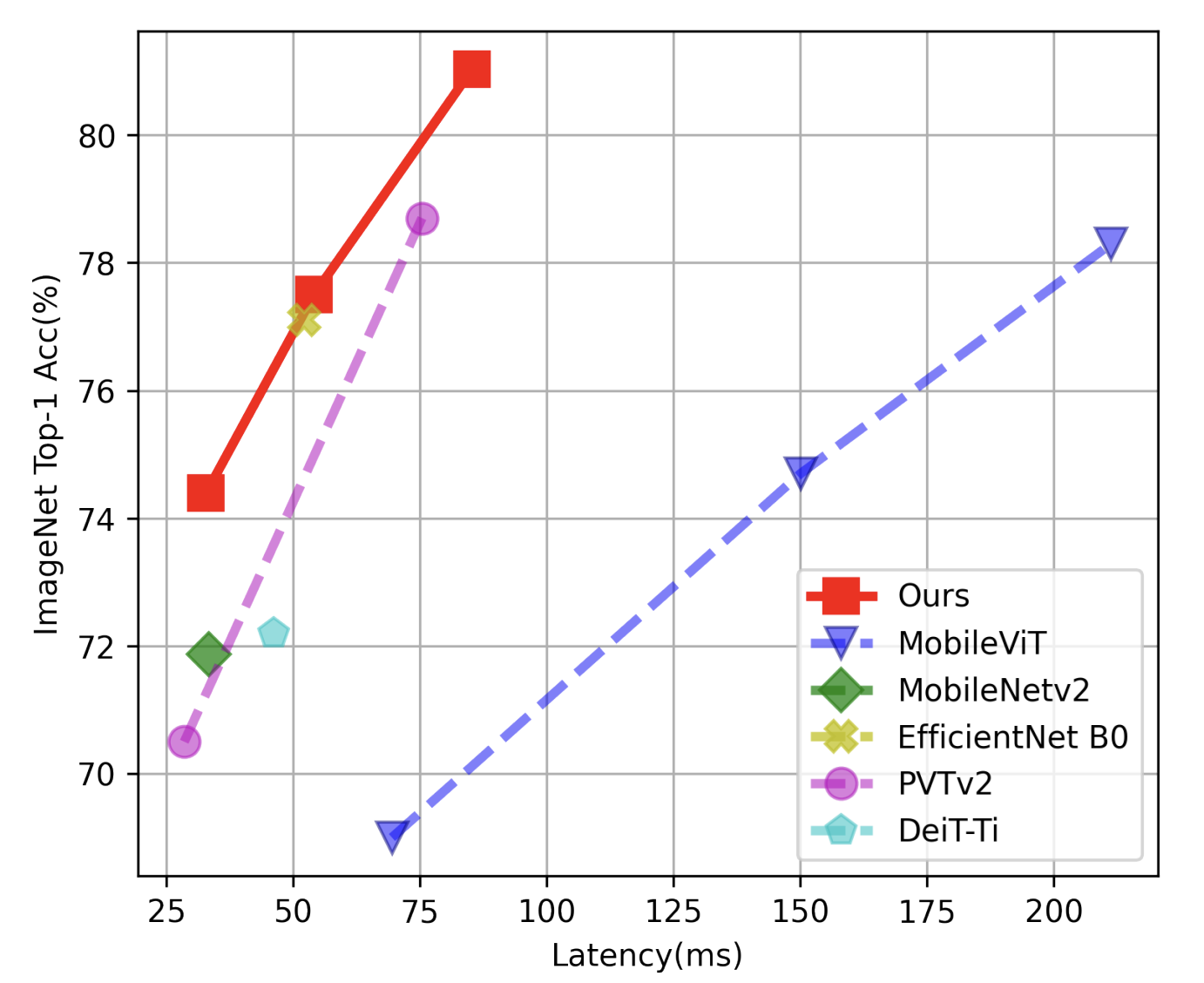 |
EdgeViTs: Competing Light-weight CNNs on Mobile Devices with Vision Transformers Junting Pan, Adrian Bulat, Fuwen Tan, Xiatian Zhu, Lukasz Dudziak, Hongsheng Li, Georgios Tzimiropoulos, Brais Martinez ECCV, 2022 [paper] [code] We introduce EdgeViTs, a new family of light-weight ViTs that for the first time, enable attention based vision models to compete with the best light-weight CNNs in the tradeoff between accuracy and on device efficiency. |
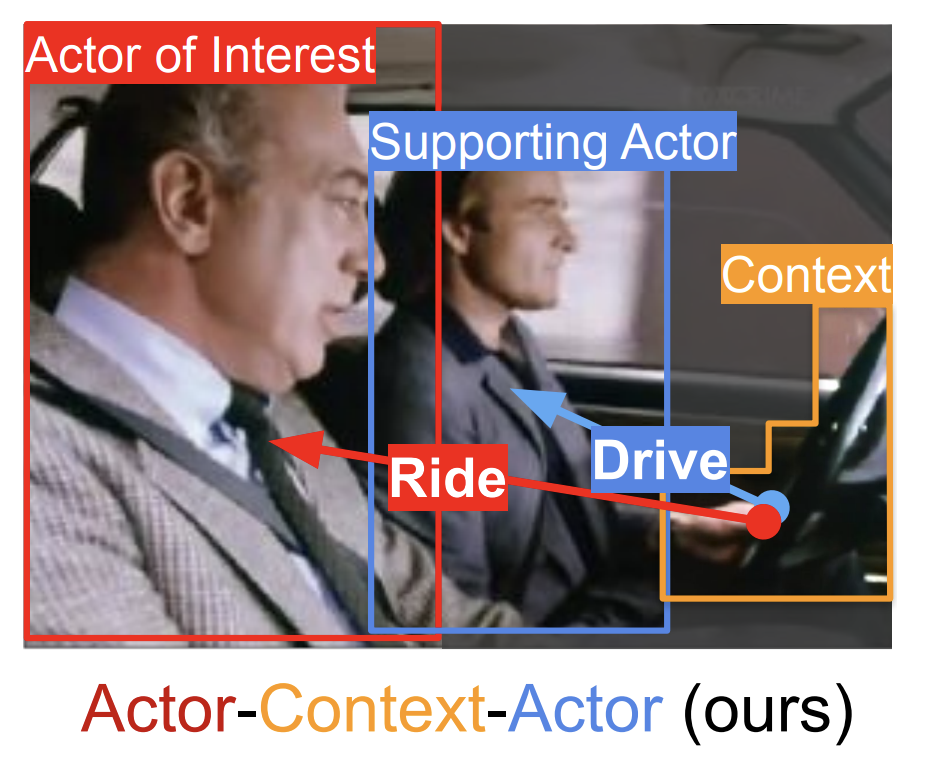 |
Actor-Context-Actor Relation Network forSpatio-Temporal Action Localization Junting Pan*, Siyu Chen*, Jing Shao, Zheng Shou, Hongsheng Li CVPR, 2021 [paper] [code] We propose to explicitly model the Actor-Context-Actor Relation, which is the relation between two actors based on their interactions with the context. Notably, our method ranks first in the AVA-Kinetics action localization task of ActivityNet Challenge 2020, outperforming other entries by a significant margin (+6.71mAP). |
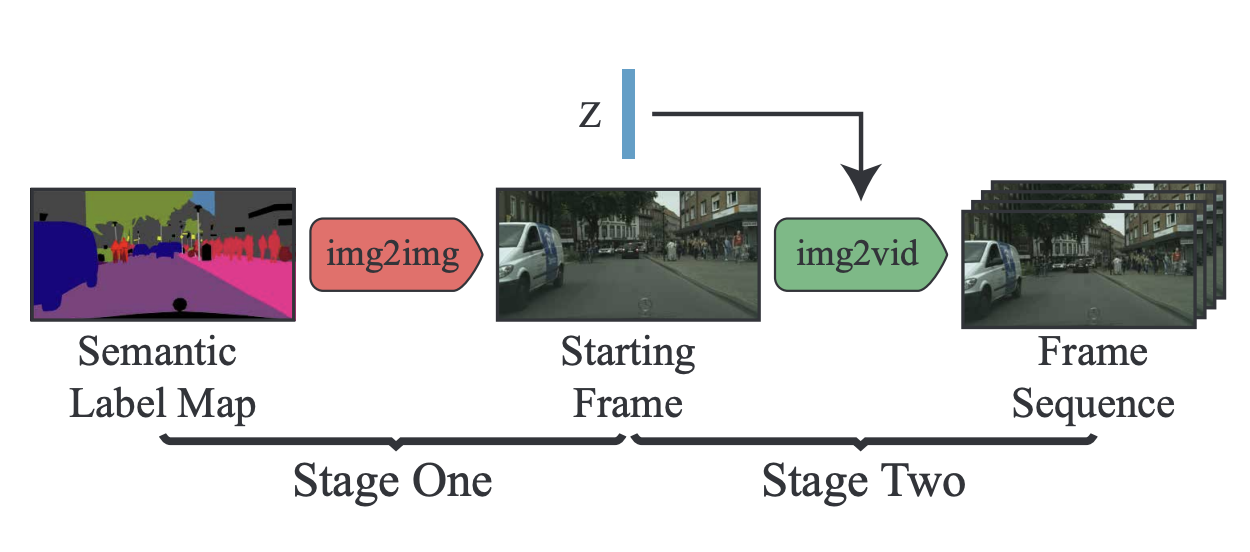 |
Video Generation from Single Semantic Label Map Junting Pan, Chengyu Wang, Xu Jia, Jing Shao, Lv Sheng, Junjie Yan, Xiaogang Wang CVPR, 2019 [paper] [code] We present a two-stage framework for video synthesis conditioned on a single semantic label map. At the first stage, we generate the starting frame from a semantic label map. Then, we propose a flow prediction network to transform the initial frame to a video sequence. |
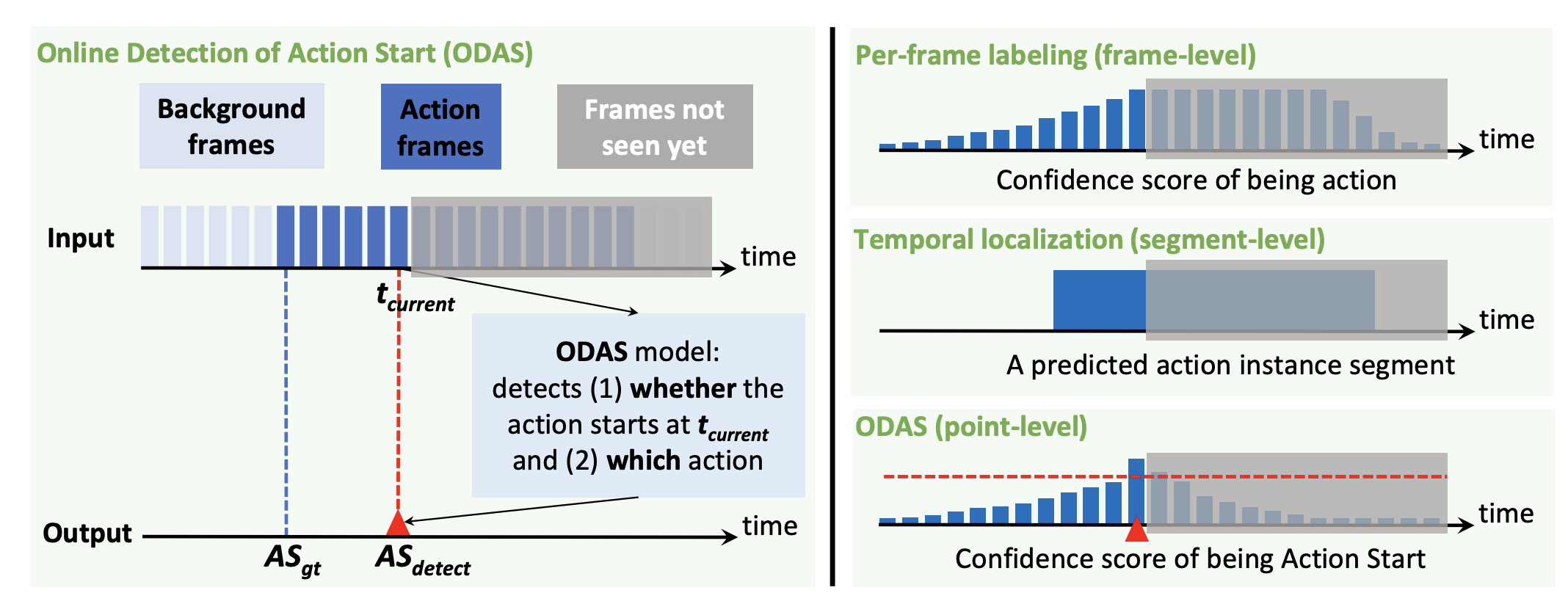 |
Online detection of action start in untrimmed, streaming videos Junting Pan*, Zheng Shou*, JJonathan Chan, Kazuyuki Miyazawa, Hassan Mansour, Anthony Vetro, Xavier Giro-i-Nieto, Shih-Fu Chang ECCV, 2018 [paper] We present a novel Online Detection of Action Start task in a practical setting involving untrimmed, unconstrained videos. Three training methods have been proposed to specifically improve the capability of ODAS models in detecting action timely and accurately. |